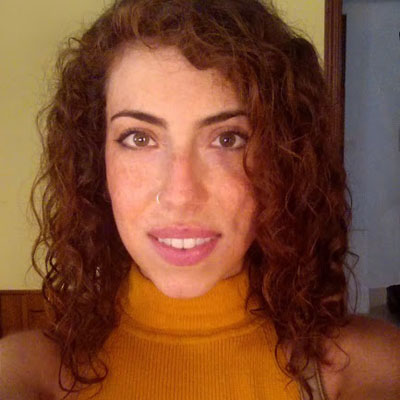
UOC, Spain
@LolaBurguenoTwitter
Artificial Intelligence (AI) has become part of everyone's life. It is used by companies to exploit the information they collect to improve the products and/or services they offer and, wanted or unwanted, it is present in almost every device around us. Lately, AI is also starting to impact all aspects of the system and software development lifecycle, from their upfront specification to their design, testing, deployment and maintenance, with the main goal of helping engineers produce systems and software faster and with better quality while being able to handle ever more complex systems. The hope is that AI will help dealing with the increasing complexity of systems and software.
There is no doubt that MDE has been a means to tame until now part of this complexity. However, its adoption by industry still relies on their capacity to manage the underlying methodological changes including among other things the adoption of new tools. To go one step further, we believe there is a clear need for AI-empowered MDE, which will push the limits of "classic" MDE and provide the right techniques to develop the next generation of highly complex model-based system and software systems engineers will have to design tomorrow.
This workshop provides a forum to discuss, study and explore the opportunities and challenges raised by the integration of AI and MDE.
We would like to address topics such as how to choose, evaluate and adapt AI techniques to Model-Driven Engineering as a way to improve current system and software modeling and generation processes in order to increase the benefits and reduce the costs of adopting MDE. We believe that AI artifacts will empower the MDE tools and boost hence the advantages, and then adoption, of MDE at industry level.
At the same time, AI is software (and complex software, in fact), we also believe that such AI-powered MDE approach will also benefit the design of AI artifacts themselves and specially to face the challenge of designing "trustable" AI software.
Last but not least, although AI is the most popular branch of computer science to create and simulate intelligence, we also believe that any kind of technique that provides human cognitive capabilities and helps creating "intelligent" software are also in the scope of this workshop. An example would be the knowledge representation techniques and ontologies that can be useful on its own or support other kinds of AI techniques.
Model-driven engineering (MDE) and artificial intelligence (AI) are two separate fields in computer science, which can clearly benefit from cross-pollination and collaboration. There are at least two ways in which such integration—which we call MDE Intelligence—can manifest:
Topics of interest for the workshop include, but are not limited to:
The 3rd edition of the MDE Intelligence workshop will be co-located with MODELS 2021 and aims to discuss current work and challenges at the intersection of MDE and AI. More information about the workshop and the programme of accepted presentations can be found here.
During the workshop, there will be a session of lightning talks around the topics that fall under the scope of the workshop.
We believe that lightning talks are a great opportunity for presenters to promote their work, to receive timely and helpful feedback and to find new collaborations. At the same, these talks will be beneficial for the workshop participants as they may broaden their knowledge and they will be able to actively participate and engage in the discussion around the presented topics.
If you are interested in presenting a lightning talk, please follow the instructions below.
Presenters will have 1-2 minutes to communicate their ideas and they can choose to use up to one slide.
We encourage the submission of proposals around the following topics:
Proposals must be submitted by email to mdeintelligence2021@easychair.org. The email should contain the presenter's name and a brief summary of the main idea behind the talk. The deadline for submitting proposals is September 30, 2021.
17:00-17:10 | Welcome |
---|---|
17:10-17:50 | Keynote by Prof. Sepp Hochreiter |
17:50-18:25 | Session 1: MDE for AI |
|
|
18:25-18:35 | Coffee break |
18:35-19:15 | Session 2: Applications |
|
|
19:15-20:00 | Lightning talks and Discussion |
|
We propose a new paradigm for deep learning by equipping each layer of a deep learning architecture with modern Hopfield networks. The new paradigm comprises functionalities like pooling, memory, and attention for each layer. Recently, we saw a renaissance of Hopfield Networks, the modern Hopfield Networks, with a tremendously increased storage capacity and converge in one update step while ensuring global convergence to local energy minimum. Surprisingly, the transformer attention mechanism is equal to modern Hopfield Networks. In layers of deep learning architectures, they allow the storage of and the access to raw input data, intermediate results, reference data, or learned prototypes. These Hopfield layers enable new ways of deep learning and provide pooling, memory, nearest neighbor, set association, and attention mechanisms. We apply deep networks with Hopfield layers to various domains, where they improved state-of-the-art on different tasks and for numerous benchmarks.
Sepp Hochreiter is heading the Institute for Machine Learning, the LIT AI Lab and the AUDI.JKU deep learning center at the Johannes Kepler University of Linz, Austria and is director of the Institute of Advanced Research in Artificial Intelligence (IARAI). Sepp Hochreiter is a pioneer of Deep Learning. His contributions the Long Short-Term Memory (LSTM) and the analysis of the vanishing gradient are viewed as milestones and key-moments of the history of both machine learning and Deep Learning. Sepp Hochreiter laid the foundations for Deep Learning in two ways. Dr. Hochreiter's seminal works on the vanishing gradient and the Long Short-Term Memory (LSTM) were the starting points for what became later known as Deep Learning. LSTM has been overwhelmingly successful in handwriting recognition, generation of writings, language modeling and identification, automatic language translation, speech recognition, analysis of audio data, as well as analysis, annotation, and description of video data.
Sepp Hochreiter is full professor at the Johannes Kepler University, Linz, Austria and head of the Institute for Machine Learning. He is a German citizen, married and has three children.
If you have questions, contact us by email at: mdeintelligence2021@easychair.org